Course 3 : Applied Statistics for DS
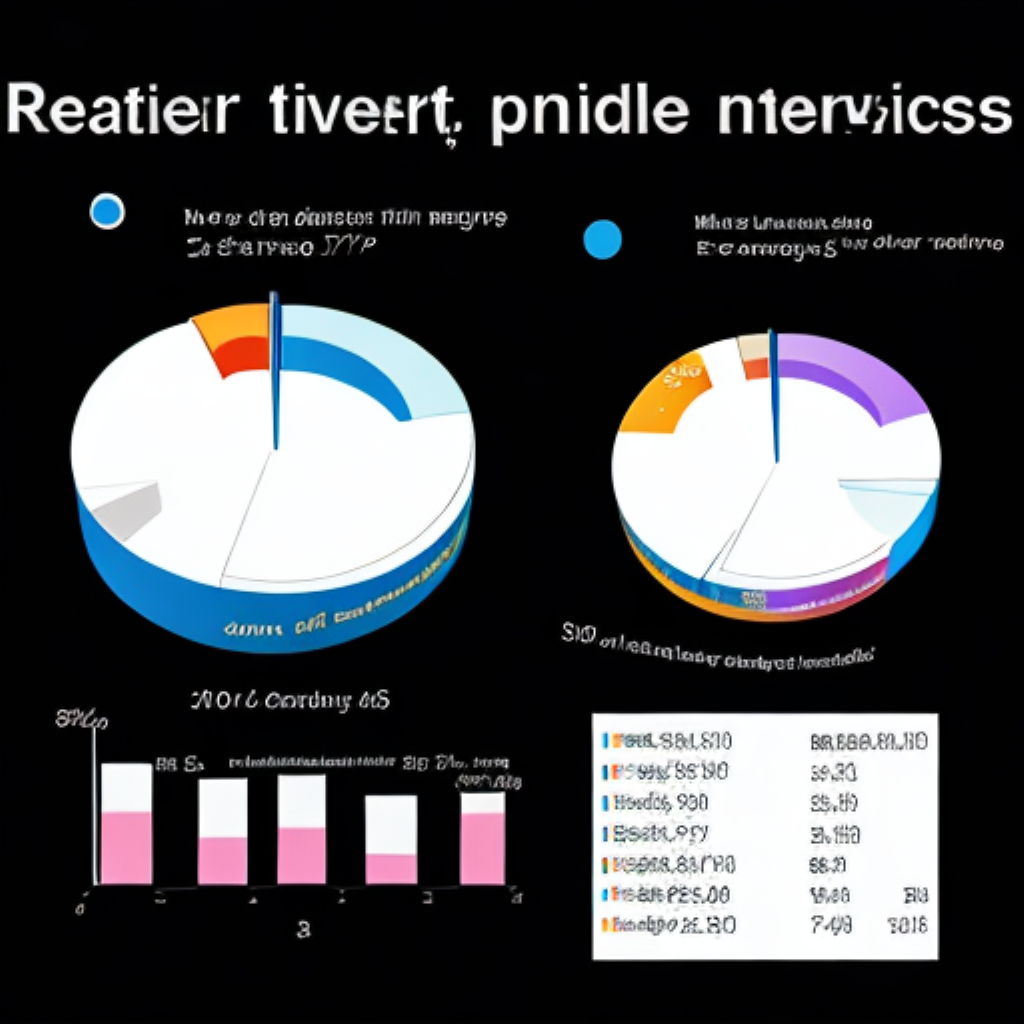
About Course
- Inferential statistics involves drawing conclusions and making predictions about a population based on a sample of data. In AI, inferential statistics play a significant role in tasks such as hypothesis testing, where statistical significance is assessed to determine the validity of observed patterns or differences in data.
- Bayesian statistics, on the other hand, provides a framework for reasoning under uncertainty by incorporating prior beliefs and updating them with observed evidence. In AI, Bayesian methods are employed in various applications such as probabilistic modeling, where uncertainties in data and predictions are explicitly quantified. For instance, in medical diagnosis systems, Bayesian statistics can be utilized to model the probability of different diseases given observed symptoms, taking into account prior knowledge about the prevalence of diseases in the population.
- Naïve Bayes is a simple yet powerful probabilistic classifier based on Bayes’ theorem with the “naive” assumption of independence between features. Despite its simplicity, Naïve Bayes is widely used in AI applications like text classification, spam filtering, and sentiment analysis. In natural language processing, Naïve Bayes classifiers are efficient and effective for tasks involving large feature spaces, such as word frequencies in documents.
Course Content
Inferential Statistics
-
Draft Lesson
Bayesian statistics – primer
Naïve Bayes for classification
Student Ratings & Reviews
No Review Yet